Putting the Power of Prognostics into the Users’ Hands
Many asset managers make decisions about their assets while being uncertain about the future. Balancing maintenance activities with production or generation planning is hindered by an inability to accurately forecast—or at least it has been in the past. Hitachi ABB Power Grids’ Asset Performance Management (APM) solution offers prognostic capabilities to let users know when to expect equipment malfunctions.
Prognostic capabilities come from collecting and connecting data from all possible sources such as databases, historians, sensors, existing Enterprise Asset Management (EAM) systems, and more. Drawing on all that data, APM uses different models, algorithms, and approaches to calculate current equipment condition to weigh risks, provide health comparisons, and tell users when to expect what sort of malfunction, and with what probability.
Getting to “when?”…and beyond
Before the introduction of prognostics, the focus was on monitoring assets and condition diagnostics: Is the equipment functional? Is there an alert/alarm? These diagnostics can be viewed like vertical drilling towards the root cause of a malfunction, asking questions like what, why, where, and how. Typically missing from such diagnostic analyses is the horizontal drilling along the timeline into the future, i.e. prognostics: When will we reach a critical condition? When can we expect a malfunction or even failure? When will we have to react?
That may sound a lot like predictive analytics, but there is more to it. A predictive solution typically provides early warnings. A data anomaly might indicate progression towards a malfunction, and that is very important information. Still, it is not telling you when you will reach that malfunction. Is it going to be today, in a week, in a month? That is what the prognostic solution addresses. On top of that, it allows the decision maker to conduct “what-if” scenario analyses.
Power of Prognostic Capabilities
Prognostic capabilities let us know when machines will fail. Plus, they give planners the tools to improve scheduling and scoping of maintenance, knowing when to do what and in which scope.
The prognostic solution helps the asset manager make more informed, and therefore better, decisions. The decision process itself has not changed necessarily. The asset managers were already weighing tradeoffs between production outage planning and maintenance scheduling, while trying to move unplanned maintenance to planned work during a low revenue period. However, prognostic capabilities change the way users think by supporting optimised, data-driven decisions informed by industry best practices and the power of machine learning.
APM, with its prognostic capabilities, is a bit like a weather app on your phone. For example, the weather app tells you there is a 60% risk of rain tomorrow and 80% risk of rain the day after that. With the prognostic solution, users can calculate the risk of, say, a bearing failure from today’s 0%, which rises to 5% next week, and 79% six weeks from now. Such a future risk profile facilitates more informed decisions. Just as you would decide whether or not to take that umbrella or plan that outdoor picnic.
The solution allows users to change the prognostic horizon from 12 days to 12 weeks and a maximum of 12 quarters or three years. The prognostic strength – a statistical calculation of confidence in the prognosis – is greater the more data we have and the larger the fleet of assets. In fact, our record prognostic horizon is six years.
Know the problems, fix the problems
In today’s world of data-driven decision making and improved machine learning, why settle for vague, non-specific predictions? Especially when it comes to your vital assets.
We worked with one company that felt it had asset management well under control so, it did not tend to react to prognostic inputs. Yet when the customer embraced the transparency created across the asset fleet, it extended the time between malfunctions from roughly 1,000 operational hours to 3,000. That is significant in terms of money as well as health and safety and risk reporting.
Hitachi ABB Power Grids’ APM with prognostic capabilities has been configured for hydro power plants, wind farms, mining, fossil power plants, and many other asset intensive industries (from discrete manufacturing to paper mills). Already the solution is configured for roughly 70 different asset types including rotating and non-rotating, mobile and stationary equipment, and ingesting binary, continuous or periodical data. This presents yet one more advantage: the user does not need to buy one solution for turbines, another for water pumps, and another for gear boxes. The entire fleet can be viewed in the dashboard of one simple, asset-agnostic solution.
With prognostic capabilities, the users can not only know there is a problem but also when in the future that problem will become a real issue. Plus, they can conduct “what-if” analyses to make the best decisions to avoid or react to that expected malfunction before it derails the company’s productivity and damages customer relationships.
That is not all. Internally, APM with prognostic capabilities helps increase connectivity and collaboration in the organisation while enabling knowledge retention, extension and dissemination. I will talk more about the relationship between human experts and the prognostic algorithms in the second article of this series.
You may be interested in:
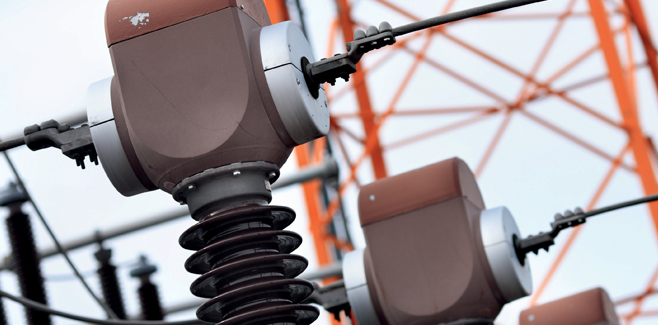
The warning that stops the storm: prediction into action
How Asset Performance Management with prognostics capabilities is changing the game for asset-intensive industries
Read more
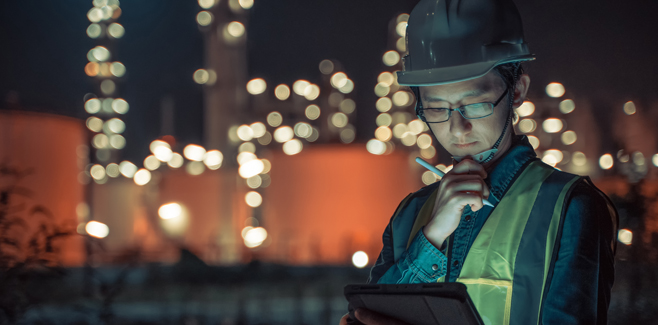
Discover the power of prognostics in Power Generation
Success in power generation is dependent on the reliability of critical assets
Read more
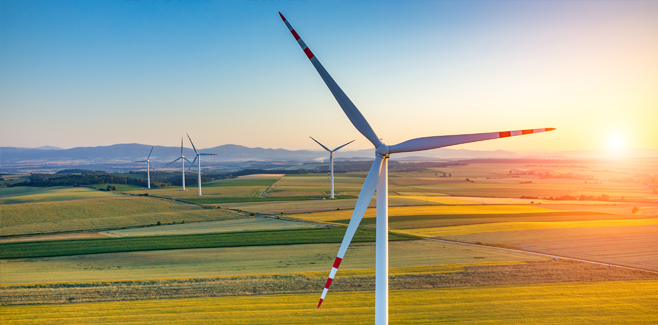
The benefit of “when” in wind energy
The entire enterprise gains from forecasting not only “what if” but also “when.”
Read more